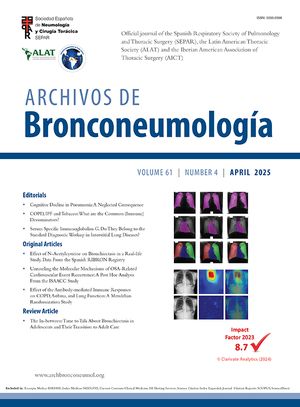
We have read with tremendous interest the letter by Accinelli and colleagues,1 where through an ecological study they determined the relationship between COVID-19 incidence and altitude in Peruvian cities. This study adds to the growing body of evidence that altitude is related to a decreased infection and mortality rate. However, there are some key points about the context of the pandemic COVID-19 in Peru that the authors did not discuss but are critical to consider before reaching conclusions.
Firstly, serological testing predominates over Polymerase Chain Reaction (PCR) tests to diagnose COVID-19 in Peruvian patients. Until 31st May, 77.91% of the COVID-19 cases in Peru were diagnosed through serological tests. Serological tests have a low sensibility and specificity for the diagnosis of COVID-19,2 and without the possibility of performing PCR tests on all patients, many may remain undiagnosed. This undiagnosed rate may be higher in altitude cities because the capital of the country and sea-level cities have most laboratories that could perform PCR tests.3
Another key point is that the data center of the health ministry has a delay in processing COVID-19 cases and deaths from all regions across the country, which explains the addition and constant update to the number of the statistics from previous months in the registry of the ministry of health.4 Furthermore, currently, the number of cases and deaths in the national registry differs from the ones according to the regional governments.4 Thus, we believe that it would be better to use regional registries in ecological studies rather than the data presented by the health ministry of Peru.
Finally, the COVID-19 pandemic in Peru did not have the same behavior in all cities. To support this information, we performed an analysis of the main cities in Peru with open data from the government,5 using new COVID-19 cases reported from May 27 to May 31. Using Farr's Law,6 I performed an exploratory analysis calculating the R1 and R2 to analyze the behavior of the pandemic in that specific moment; we classified the pandemic stage as: A or B if the pandemic was accelerating; C if the had reached the peak (considered as R1 and R2 between 0.8 and 1.2); D or E if the pandemic was decelerating in that city. As can be seen in Table 1, all regions had different ratios meaning they were at different stages of the pandemic. In a cross-sectional analysis as performed by Accinelli and colleagues,1 the different stages of the pandemic across Peru would be seen as a relationship between altitude and the incidence of COVID-19 cases but performing an analysis with Farr's Law provides enough information to refute this hypothesis. The spurious correlation found by the mentioned study could be the result of the better accomplishment of the quarantine of these cities during the early days of this pandemic. Finally, it is important to remember that ecological studies, such as the one conducted by Accinelli et al, do not consider many other factors that play a role in the incidence of COVID-19; furthermore, they are prone to “ecological fallacy”, where the inferences made with aggregated data are contradictory to those obtained through individual data.7
Epidemic stages according to Farr's Law in Peruvian regions.
Region | R1 and R2 | Value | Epidemic stage | Basic interpretation |
---|---|---|---|---|
>1500 mamsl of altitude | ||||
PASCO | R1 | 0.85 | D | Deceleration |
R2 | 3.55 | |||
JUNIN | R1 | 0.76 | E | Deceleration |
R2 | 0.84 | |||
PUNO | R1 | 0.73 | E | Deceleration |
R2 | 0.56 | |||
HUANCAVELICA | R1 | 0.71 | D | Deceleration |
R2 | 1.40 | |||
CUSCO | R1 | 1.24 | B | Acceleration |
R2 | 0.27 | |||
ANCASH | R1 | 1.07 | B | Acceleration |
R2 | 0.78 | |||
AYACUCHO | R1 | 0.57 | D | Deceleration |
R2 | 5.30 | |||
CAJAMARCA | R1 | 1.60 | B | Acceleration |
R2 | 0.56 | |||
APURIMAC | R1 | 1.13 | B | Acceleration |
R2 | 0.89 | |||
AMAZONAS | R1 | 1.30 | B | Acceleration |
R2 | 0.89 | |||
AREQUIPA | R1 | 1.00 | D | Deceleration |
R2 | 0.55 | |||
HUANUCO | R1 | 0.75 | E | Deceleration |
R2 | 0.40 | |||
<1500 mamsl of altitude | ||||
MOQUEGUA | R1 | 0.44 | D | Deceleration |
R2 | 1.58 | |||
SAN MARTIN | R1 | 1.02 | C | Peak of the curve |
R2 | 1.19 | |||
TACNA | R1 | 3.09 | B | Acceleration |
R2 | 0.65 | |||
ICA | R1 | 0.63 | E | Deceleration |
R2 | 0.58 | |||
LIMA | R1 | 0.84 | C | Peak of the pandemic |
R2 | 0.97 | |||
UCAYALI | R1 | 1.19 | C | Peak of the curve |
R2 | 1.01 | |||
MADRE DE DIOS | R1 | 2.00 | B | Acceleration |
R2 | 0.22 | |||
LORETO | R1 | 0.56 | D | Deceleration |
R2 | 1.06 | |||
PIURA | R1 | 0.82 | C | Peak of the curve |
R2 | 0.90 | |||
LAMBAYEQUE | R1 | 0.62 | D | Deceleration |
R2 | 2.17 | |||
LA LIBERTAD | R1 | 1.71 | B | Acceleration |
R2 | 0.63 | |||
TUMBES | R1 | 1.84 | B | Acceleration |
R2 | 0.37 | |||
CALLAO | R1 | 1.00 | E | Deceleration |
R2 | 0.70 |
Ministerio de Salud. n.d. “Fallecidos Por COVID-19.” Plataforma Nacional de Datos Abiertos. Peru. https://www.datosabiertos.gob.pe/dataset/fallecidos-por-covid-19-ministerio-de-salud-minsa.
JPC participated in conceptualization, data collection; writing – original draft; writing – review & editing, reviewed the final manuscript and accepted all responsibility for the information provided.
FundingThis study was funded by the authors.
Conflict of interestJPC declare to have no conflict of interest.