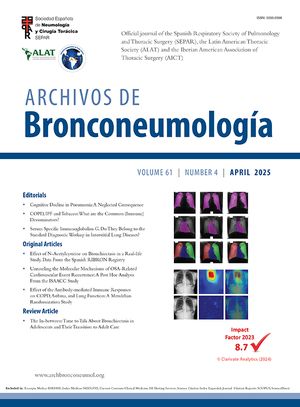
Early diagnosis of lung cancer (LC) is crucial to improve survival rates. Radiomics models hold promise for enhancing LC diagnosis. This study assesses the impact of integrating a clinical and a radiomic model based on deep learning to predict the malignancy of pulmonary nodules (PN).
MethodologyProspective cross-sectional study of 97 PNs from 93 patients. Clinical data included epidemiological risk factors and pulmonary function tests. The region of interest of each chest CT containing the PN was analysed. The radiomic model employed a pre-trained convolutional network to extract visual features. From these features, 500 with a positive standard deviation were chosen as inputs for an optimised neural network. The clinical model was estimated by a logistic regression model using clinical data. The malignancy probability from the clinical model was used as the best estimate of the pre-test probability of disease to update the malignancy probability of the radiomic model using a nomogram for Bayes’ theorem.
ResultsThe radiomic model had a positive predictive value (PPV) of 86%, an accuracy of 79% and an AUC of 0.67. The clinical model identified DLCO, obstruction index and smoking status as the most consistent clinical predictors associated with outcome. Integrating the clinical features into the deep-learning radiomic model achieves a PPV of 94%, an accuracy of 76% and an AUC of 0.80.
ConclusionsIncorporating clinical data into a deep-learning radiomic model improved PN malignancy assessment, boosting predictive performance. This study supports the potential of combined image-based and clinical features to improve LC diagnosis.