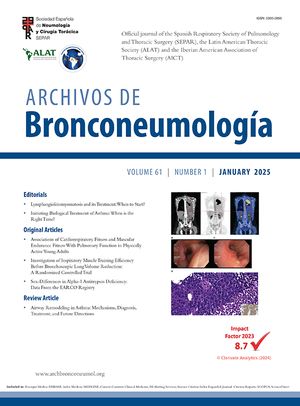
CURRENT SITUATION AND LONG-TERM CONSEQUENCES OF COVID-19 INFECTION
More infoThe COVID-19 pandemic created tremendous challenges for health-care systems. Intensive care units (ICU) were hit with a large volume of patients requiring ICU admission, mechanical ventilation, and other organ support with very high mortality. The Centro de Investigación Biomédica en Red-Enfermedades Respiratorias (CIBERES), a network of Spanish researchers to investigate in respiratory disease, commissioned the current proposal in response to the Instituto de Salud Carlos III (ISCIII) call.
MethodsCIBERESUCICOVID is a multicenter, observational, prospective/retrospective cohort study of patients with COVID-19 admitted to Spanish ICUs. Several work packages were created, including study population and ICU data collection, follow-up, biomarkers and miRNAs, data management and quality.
ResultsThis study included 6102 consecutive patients admitted to 55 ICUs homogeneously distributed throughout Spain and the collection of blood samples from more than 1000 patients. We enrolled a large population of COVID-19 ICU-admitted patients including baseline characteristics, ICU and MV data, treatments complications, and outcomes. The in-hospital mortality was 31%, and 76% of patients required invasive mechanical ventilation. A 3-6 month and 1 year follow-up was performed. Few deaths after 1 year discharge were registered. Low anti-SARS-CoV-2 S antibody levels predict mortality in critical COVID-19. These antibodies contribute to prevent systemic dissemination of SARS-CoV-2. The severity of COVID-19 impacts the circulating miRNA profile. Plasma miRNA profiling emerges as a useful tool for risk-based patient stratification in critically ill COVID-19 patients.
ConclusionsWe present the methodology used in a large multicenter study sponsored by ISCIII to determine the short- and long-term outcomes in patients with COVID-19 admitted to more than 50 Spanish ICUs.
La pandemia de COVID-19ha supuesto un enorme reto para los sistemas sanitarios. Las unidades de cuidados intensivos (UCI) se han visto afectadas por un gran volumen de pacientes que requerían ingreso en la UCI, ventilación mecánica y otras asistencias de órganos con gran mortalidad. El Centro de Investigación Biomédica en Red-Enfermedades Respiratorias (CIBERES), una red de investigadores españoles para el estudio de enfermedades respiratorias, encargó la presente propuesta en respuesta a la convocatoria del Instituto de Salud Carlos III (ISCIII).
MétodosCIBERESUCICOVID es un estudio de cohortes multicéntrico, observacional, prospectivo/retrospectivo de pacientes con COVID-19 ingresados en UCI españolas. Se crearon varios paquetes de trabajo: población de estudio y recogida de datos en la UCI, seguimiento, biomarcadores y miRNA, gestión de datos y calidad.
ResultadosEste estudio incluyó a 6.102 pacientes consecutivos ingresados en 55 UCI distribuidas homogéneamente por toda España, y se recogieron muestras de sangre de más de 1.000 pacientes. Se incluyó a una amplia población de pacientes ingresados en UCI de COVID-19, y se registraron las características basales, los datos de la UCI y la ventilación mecánica, las complicaciones de los tratamientos y los resultados. La mortalidad hospitalaria fue del 31%, y el 76% de los pacientes requirieron ventilación mecánica invasiva. Se realizó un seguimiento de 3-6 meses y de 1 año. Se registraron pocas muertes después del alta a 1 año. Las bajas cifras de anticuerpos anti-SARS-CoV-2 S predicen la mortalidad en la COVID-19 crítica. Estos anticuerpos contribuyen a prevenir la diseminación sistémica del SARS-CoV-2. La gravedad de la COVID-19 influye en el perfil de miRNA circulantes. El perfil de miRNA plasmático emerge como un dato útil para la estratificación basada en el riesgo de los pacientes con COVID-19 en estado crítico.
ConclusionesSe presenta la metodología utilizada en un gran estudio multicéntrico patrocinado por el ISCIII para determinar los resultados a corto y largo plazo en pacientes con COVID-19 ingresados en más de 50 UCI españolas.
The COVID-19 pandemic, which started in early 2020, created tremendous challenges for health-care systems. Intensive care units (ICU) were hit with a large volume of patients requiring ICU admission, mechanical ventilation, and other organ support with very high mortality (30%–50%).1,2 Although we have improved disease management, the acute mortality of patients admitted to ICU remains high.3,4 In March 2020, the Instituto de Salud Carlos III (ISCIII) called for Spanish investigators to perform studies in 2020–2021 to improve the knowledge and management of COVID-19. The Centro de Investigación Biomédica en Red-Enfermedades Respiratorias (CIBERES), a network of Spanish researchers funded by ISCIII to investigate in respiratory disease, commissioned the current proposal in response to the ISCIII call.
The most urgent problems early in the pandemic centered on patients admitted to ICU. Therefore, we decided to focus on these severely ill patients and presented an ambitious project to collect sequential clinical, physiological, and biological data from more than 5000 patients in more than 50 Spanish ICUs. In addition, we planned to collect plasma samples of more than 1000 patients admitted to those ICUs. The project was granted and started on May 1st, 2020, with three main objectives. First, we aimed to determine the epidemiology and prognostic factors for patients with COVID-19 admitted to Spanish ICUs. Second, we wanted to determine the inflammatory patterns and epigenetic factors associated with different prognoses. Finally, we wanted to follow-up all patients discharged from ICUs at 3, 6, and 12 months to determine the consequences and mortality associated with COVID-19. We achieved all planned objectives and published several manuscripts for each one.
In the current article, we describe the methodology of the CIBERESUCICOVID study, focusing on organization, data collection, and purification.
Material and methodsStudy designCIBERESUCICOVID is a multicenter, observational, prospective/retrospective cohort study of patients with COVID-19 admitted to Spanish ICUs (Table 1). Our institution's internal review board (Comité Ètic d’Investigació Clínica, no. HCB/2020/0370) approved the study, and a member of the study team contacted local researchers, with participating hospitals obtaining local ethics committee approval as needed. Results were reported in accordance with the Strengthening the Reporting of Observational Studies in Epidemiology (STROBE) guidelines.
List of participating centers.
Clínica Sagrada Família |
Complexo Hospitalario Universitario de Ourense |
HM Hospitales Madrid |
Hospital Álvaro Cunqueiro |
Hospital Clínic Barcelona |
Hospital Clínic Universitari de València |
Hospital Clínico Universitario de Santiago |
Hospital Clínico Universitario de Valladolid |
Hospital de León |
Hospital de Mataró |
Hospital de Santa Maria |
Hospital de Tortosa Verge de la Cinta |
Hospital del Mar |
Hospital General de Segovia |
Hospital General Río Carrión |
Hospital General Universitario Gregorio Marañón |
Hospital Germans Trias i Pujol |
Hospital Infanta Leonor de Madrid |
Hospital La Fe de Valencia |
Hospital Nuestra Señora de Gracia |
Hospital Parc Taulí |
Hospital Punta de Europa-Algeciras |
Hospital Sagrat Cor |
Hospital San Juan de Dios |
Hospital San Pedro de Alcántara |
Hospital Son Llàtzer |
Hospital Universitari Arnau de Vilanova |
Hospital Universitari de Bellvitge |
Hospital Universitari Joan XXIII de Tarragona |
Hospital Universitari Mútua de Terrassa |
Hospital Universitari Son Espases |
Hospital Universitario 12 de Octubre |
Hospital Universitario Central de Asturias |
Hospital Universitario de Basurto |
Hospital Universitario de Cruces |
Hospital Universitario de Getafe |
Hospital Universitario de Gran Canaria Doctor Negrín |
Hospital Universitario de Jerez de la Frontera |
Hospital Universitario de la Princesa |
Hospital Universitario de Móstoles |
Hospital Universitario de Salamanca |
Hospital Universitario de Torrejón |
Hospital Universitario La Paz |
Hospital Universitario Lucus Augusti |
Hospital Universitario Marqués de Valdecilla |
Hospital Universitario Príncipe de Asturias |
Hospital Universitario Ramón y Cajal |
Hospital Universitario Reina Sofia, Córdoba |
Hospital Universitario Río Hortega |
Hospital Universitario San Agustín |
Hospital Universitario Sant Joan d’Alacant |
Hospital Universitario Vall d’Hebron |
Hospital Virgen de Valme |
Hospital Virgen del Rocío |
Hospital Virgen Macarena |
Data collection started in May 2020, and we collected, anonymized, stored, and managed all data via the REDCap (Research Electronic Data Capture) tool, hosted at CIBER, Spain. Trained local researchers incorporated data from patients’ medical records into a separate database. We appointed a steering committee that met every week, and we created several work packages.
Study population and ICU data collectionAll consecutive patients admitted to participating ICUs from February 25th to February 28th, 2021, were enrolled if they fulfilled the following criteria:≥18 years old, ICU admission, and laboratory confirmed SARS-CoV-2 infection. Patients were excluded if they were admitted to an ICU for other reasons. After enrollment, we collected data on demographics, comorbidities, clinical symptoms, disease chronology, and treatment administered at hospital admission.
The site researchers subsequently collected data acquired at hospital admission, ICU admission, start of mechanical ventilation, 72-96 h after ICU admission, weaning off mechanical ventilation, ICU discharge, and hospital discharge. This included vital signs, respiratory support devices, adjunctive therapies, laboratory findings, and arterial blood gases. We studied hemodynamic parameters and organ dysfunction using the Sequential Organ Assessment Failure Score (SOFA) at ICU admission. We also collected data on pharmacological treatments and interventions administered at and during ICU admission until either ICU or hospital discharge or death. Mechanical ventilation settings were collected if used, and other pulmonary mechanical variables (e.g., driving pressure or static compliance of the respiratory system) were calculated. Impairment in oxygenation was analyzed using the PaO2/FiO2 ratio, and abnormalities of CO2 metabolism were studied using the ventilatory ratio (VR), a surrogate parameter of Vd/Vt.5 The worst event values were recorded preferentially.
The reported outcomes included in-hospital mortality, ICU mortality, 28-day mortality, 90-day mortality, duration of ventilation, and lengths of stay in ICU and hospital. ICU- and ventilator-free days were also computed as previously reported.6 All the pulmonary and extrapulmonary complications during ICU admission were also collected and included at hospital discharge.
Follow-up at 3, 6, and 12 monthsGeneral symptoms, respiratory symptoms, quality of life, and survival status were assessed at 3, 6, and 12 months after hospital discharge either during a home visit or phone call. Patients were asked about cardiovascular or infectious complications, emergency department visits, or hospital readmission. Post-COVID syndrome was defined according to the suggestion of the World Health Organization7 as the presence of symptoms such as fatigue, shortness of breath, and cognitive dysfunction that affect daily quality of life 3 months after probable or confirmed SARS-Cov2 infection, without being explained by other alternative diagnoses.
Airway function (spirometry, lung volume, and diffusing capacity) was measured according to the guidelines of the American Thoracic Society.8 Pulmonary parameters included forced vital capacity (FVC), forced expiratory volume in the first second (FEV1), the FEV1/FVC ratio, and lung diffusing capacity for carbon monoxide. The results were expressed as a percentage of the predicted value according to the European Community Lung Health Survey.9 A chest x-ray or computed tomography (CT) scan was also performed.
The 12-Item Short Form Survey (SF-12) was used to assess quality of life across eight health domains (general health, physical functioning, role physical, body pain, vitality, social functioning, role emotional, and mental health). This questionnaire has been validated in healthy populations, in several chronic diseases and conditions, and in acute respiratory distress syndrome (ARDS) survivors.10-12 The standardized SF-12 is described in means±standard deviation according to the normative standards established by Ware et al.,13 such that persons with a normal health-related quality of life have an average SF-12 score of 50±10.
Blood samplesWe collected a 5mL blood sample in the first 48 h of ICU admission for COVID-19 infection, obtained 2mL of plasma from EDTA tubes after centrifugation at the recruiting center, and stored the sample at –80°C. Plasma samples were received centrally at the BioSepsis laboratory of Hospital Universitario Río Hortega de Valladolid/IBSAL, from where they were further split into three aliquots. The first was shipped to the laboratory of the National Center of Microbiology (Majadahonda, Spain) to profile anti-SARS-CoV-2 antibodies. The second aliquot was shipped to the IRB-Lleida (Spain) for microRNA profiling. The third remained at the BioSepsis group for biomarker profiling, antigenemia evaluation, and viral load quantification.
BiomarkersTo identify biomarkers associated with an increased risk of mortality on ICU admission, we profiled 40 biomarkers using the Ella-SimplePlex™ system from Biotechnne (Lipocalin-2/NGAL, ICAM-1, Myeloperoxidase, VCAM-1/CD106, interleukin 1 beta, B7-H1/PD-L1, G-CSF, IFN-β, D-dimer, ferritin, E-selectin, RANTES, angiopoietin 2, GM-CSF, IL-7, IL-1ra, IL-6, IL-10, IL-17A, CXCL10/IP10, CCL2, granzyme B, IFN-γ, IL-12p70, IL-15, TNF-α, IL-2, IL-4, IFN-α, granzyme A, IL-8, endothelin-1, CD27, FAS, SPD, pentraxin 3, TREM-1, EGF, UPA-1, CTLA-4). This measured endothelial dysfunction, neutrophil degranulation, coagulation activation, neutrophil activation, T cell and monocyte chemotaxis, interferon response, and Th1, Th2, and TH17 response. Viral RNA was quantified in plasma by droplet-digital polymerase chain reaction (PCR) using the Bio-Rad SARS-CoV-2 ddPCR kit on a QX-200 ddPCR platform, according to manufacturer's specifications. The presence of N antigen of SARS-CoV-2 in plasma was assessed using the Panbio COVID-19 Ag Rapid Test Device (Abbott). We used a specific immunoassay developed by our team to quantify anti-SARS-CoV-2 S IgG and IgM antibodies.14
Quantification of microRNARNA isolation and microRNA (miRNA) quantification were conducted in the same laboratory using standardized protocols. Experienced staff blinded to patient data performed the miRNA quantification. Total RNA was isolated from frozen plasma using the miRNeasy Serum/Plasma Advanced kit (Qiagen, Germany) according to the manufacturer's instructions. For normalization, synthetic Caenorhabditis elegans miR-39-3p (cel-miR-39-3p) was added as an external reference miRNA. The mixture was supplemented with MS2 carrier RNA (Roche Diagnostics, Germany). The RNA Spike-In Kit (Qiagen, Germany) was added to monitor RNA isolation. RNA was eluted with RNAse-free water and stored at –80°C.
Reverse transcription (RT) was performed using the miRCURY LNA RT Kit (Qiagen, Germany). An additional spike-in UniSp6 (Qiagen, Germany) was added to monitor the RT reaction and complementary DNA was stored at 20°C. The miRNAs were analyzed using miRCURY LNA miRNA Custom Panels (384-well plates) (Qiagen, Germany). qPCR was carried out using the QuantStudioTM 7 Flex Real-Time PCR System (Applied Biosystems, USA). Synthetic UniSp3 was analyzed as an interplate calibrator and qPCR control. Amplification curves were evaluated using QuantStudio Software v1.3 (Thermo Fisher Scientific, USA).
To ensure optimal data quality, the spike-in RNA templates were first analyzed to monitor RNA extraction procedure uniformity and RT and PCR efficiency. Hemolysis contamination was excluded, as previously described. We considered miRNAs expressed at cycle quantification (Cq) values of<35. Relative quantification was performed using the 2-ΔCq method (ΔCq=CqmiRNA-Cqcel-miR-39-3p). Expression levels were log-transformed for statistical purposes. This protocol has been adapted for bronchial aspirates.15
Data managementCIBERESUCICOVID used REDCap as a single source of truth for data management. A second database was created to facilitate data analysis and exploitation, which was populated from REDCap through a periodic automated process. During this process, data were retrieved from REDCap forms in a specific order, units of measurement were unified, and variables were converted as necessary. Due to the number of patients and the amount of data, this process was parallelized for periodic completion. All data were stored in encrypted servers within the EU, and all data access was recorded and limited to authorized researchers. This architecture is further detailed elsewhere.16
Automated analysis and sub-studiesMost analyses were automated to maximize scalability and reproducibility. The system could generate univariate analyses (e.g., basic statistics and statistical tests) and multivariate analyses (e.g., correlations and regressions), and survival curves (e.g., Kaplan-Meier), among others, by completing a short configuration (e.g., population restrictions and variables of interest). This automatization allowed us to iterate continuously in response to the many hypotheses proposed by medical experts in sub-studies, generating quick results with minimal overhead while ensuring reproducibility.
The wide scope and size of the CIBERES-UCI-COVID project enabled many derived sub-studies to emerge. To optimize time and resources, sub-studies went through several stages:
- 1.
Definition of topic and responsible person.
- 2.
Approval by project committee.
- 3.
Definition of target population and variables of interest. This step produced a first set of tables with basic statistics and univariate test results that could be iterated upon based on the criteria of the responsible person.
- 4.
Variable refinement (addition, deletion, modification) and multivariate analysis. This step was tailored for each sub-study and could include one or more analysis (e.g., population clustering, survival analysis, or propensity score analysis).
Valid scientific conclusions require the input of high-quality data with an excellent data quality assurance (DQA) process during all study phases (Table 2).
List of tasks performed with our DQA tool.
Missing data | The identification of the core variables that constitute the minimum data set (MDS) of each record |
---|---|
Create clinical impact variables | Creation of clinical variables like APACHE-II, SOFA, PaFi, BMI, CURB65, compliance, driving pressure, ventilation ratio, etc. |
Unstructured data | Rule programming to transform unstructured text to standardized encoded data. Use of specific lists or international classification systems such as ICD-10 for diseases or ATC for drugs |
Outliers | Development of rules to identify and validate extreme values |
Data inconsistencies | Development of rules to identify and manage inconsistencies related to dates, events, duration of treatments, etc. |
Database homogenization | Development of rules to automatize the homogenization of the different laboratory units of the analytical variables |
Tracking report | Weekly sample tracking report to monitor the data inclusion |
Dashboard | To monitor, in real time, the different existing data inconsistencies |
Combined filter | To identify queries associated with the sub-studies (patient profile) and/or desired variables associated with a working hypothesis |
Dashboard for queries management | Mass sending of queries, visualization of data entry responses in RedCap, internal resolution of queries, and automatic closing of inconsistencies when the system detects that they have disappeared |
SPSS database | Dump of data in SPSS format, weekly, after cleaning and debugging data |
During data collection, we designed a parallelization strategy for data collection and cleaning. This used a custom-built DQA tool (based on the NAIHA medical device) that facilitated real-time communication using an application programming interface (API) with the study's electronic case report form (REDCap) (Fig. 1). This phase sought to monitor the data, generate reports, identify inconsistencies or missing data, and manage queries. The good design made it possible to develop the norms and rules to automate these tasks.
During data analysis, we grouped all collected data by the working hypotheses or associated sub-studies for specific research. The data analysis module allowed the creation of filters to classify the ongoing sub-studies and to manage them independently. This way, we could manage the more than 5 million existing data points in an intuitive and efficient way, having only the necessary subjects and variables for each sub-study. This module personalized the query creation and sending, avoiding unnecessary requests being sent to the researchers.
ResultsStudy population and ICU dataThe electronic case report form collected information on demographic data, comorbidities, and disease chronology, including treatments, interventions, outcomes, and complications during the hospital stay, with detailed clinical information on the most significant events, such as admission and discharge from the ICU or the start of mechanical ventilation. In total, the study included 55 Spanish ICUs in 13 autonomous communities, from which we included 8921 patients admitted consecutively to ICU with COVD-19 (Fig. 2). Of these, we obtained complete information on 6102 patients (70.3% men), exceeding the initial goal of 5000 patients, with a mean age of 63 (54-71) years. The most common comorbidities were arterial hypertension (50.4%) and diabetes mellitus (24.7%). On ICU admission, the APACHE-II and SOFA scores were 12 (9-16) and 5 (4-7), respectively. Also of note, 76% required invasive mechanical ventilation, increasing in-hospital mortality from 31% to 37% (Fig. 3). The average ICU stay was 13 (7-27) days and the average hospital stay was extended to 23 (14-40) days. Table 3 shows the demographic and clinical characteristics of these patients.
Demographic and clinical characteristics of patients with COVID-19 admitted to Spanish ICUs.
All patients, n | 6102 |
Age (years) | 63 [54-71] |
Sex (male) | 4290 (70.3) |
BMI | 28.8 [26.0-32.3] |
Comorbidities (No. with data) | |
Hypertension | 3075 (50.4) |
Diabetes mellitus | 1507 (24.7) |
Chronic cardiovascular disease | 793 (13.0) |
Chronic pulmonary disease | 622 (10.2) |
Chronic kidney disease | 439 (7.2) |
Disease chronology from symptoms onset | |
To hospital admission (days) | 7 [5-9] |
To ICU admission (days) | 9 [7-12] |
To IMV start (days) | 10 [7-13] |
Symptoms at hospital admission | |
Fever | 5123 (82.9) |
Dry cough | 3740 (61.3) |
Productive cough | 790 (12.9) |
Shortness of breath | 4432 (72.6) |
Anosmia | 442 (7.2) |
Headache | 834 (13.7) |
Myalgia | 1646 (27.0) |
Diarrhea | 1320 (21.6) |
Severity scores at ICU admission | |
SOFA | 5 [4-7] |
APACHE II | 12 [9-16] |
Vital signs at ICU admission | |
Temperature (°C) | 36.8 [36.0-37.5] |
Mean arterial pressure (mmHg) | 88 [75-100] |
Respiratory rate (bpm) | 25 [21-31] |
Blood gases and laboratory parameters at ICU admission | |
PaO2/FiO2 (mmHg) | 112.5 [80.6-163.3] |
PaCO2 (mmHg) | 39.0 [34.0-47.0] |
pH | 7.41 [7.34-7.46] |
Lymphocyte count (×109/L) | 0.69 [0.47-0.99] |
D-dimer (ng/mL) | 1000.0 [518.0-2334.75] |
C-reactive protein (mg/L) | 128.0 [61.5-220.0] |
Ferritin (pg/mL) | 1142.0 [605.7-1880.3] |
IL-6 (pg/mL) | 86.6 [31.6-222.3] |
Interventions | |
IMV | 4638 (76.0) |
Prone position | 3667 (60.1) |
Tracheostomy | 1812 (29.7) |
ECMO | 164 (2.7) |
Renal replacement therapy | 549 (9.7) |
Complications | |
ARDS | 4792 (78.6) |
Bacterial pneumonia | 1861 (30.5) |
Pulmonary embolism | 568 (9.3) |
Coagulation disorder | 1458 (23.9) |
Acute renal injury | 1953 (32.0) |
Liver disfunction | 1825 (29.9) |
Outcomes | |
ICU length (days) | 13 [7-27] |
IMV length (days) | 15 [8-27] |
Hospital length (days) | 23 [14-40] |
In-hospital mortality | 1911 (31.4) |
APACHE-II: Acute Physiology and Chronic Health Evaluation; ARDS: acute respiratory distress syndrome; BMI: body mass index; ECMO: extracorporeal membrane oxygenation; ICU: intensive care unit; IL-6: interleukin-6; IMV: invasive mechanical ventilation; SOFA: Sequential Organ Failure Assessment.
Data are expressed as n (%) or median [interquartile range].
Of 3367 patients who survived hospitalization, 2382 completed a full 12-month follow-up period. During this period, 10% visited the emergency department and 1% died (17). At 12-months, spirometry data were available for 698 patients, lung diffusing capacity for carbon monoxide data for 581, and CT scan data for 602 (Fig. 4).
Blood samplesA total of 1338 samples were collected from 26 ICUs throughout Spain and screened for whether they met the inclusion and clinical data quality criteria. Finally, 1068 samples were processed, fractioned, and distributed according to the methods.
BiomarkersThe CIBERESUCICOVID project pioneered in revealing the association of the plasma viral RNA load and antigenemia with critical illness and mortality.14,18 The project also demonstrated that the presence of viral RNA in plasma can be detected using standard real-time PCR methods.19 We described the association between plasma viral RNA load and the presence of a dysregulated host response to the infection, mimicking that occurring in sepsis.18 Furthermore, we described that low levels of anti-SARS-CoV-2 S antibodies were associated with mortality and the dissemination of viral components to the blood.14 These results led to two opinion papers,20,21 contributed to a review paper on COVID-19 pathogenesis,22 and to the submission of a patent on the use of antigenemia to predict mortality to the European Patent Office (EP20383140). Finally, using clinical follow-up data from survivors at 3-, 6-, and 12-month following hospital discharge, we can identify biomarkers linked to severity and outcomes in the acute phase, including the development of long COVID-19 symptoms in these patients. Research is ongoing.
Quantification of microRNAIn a preliminary study, we analyzed the circulating miRNA profile of patients admitted to ICU and in patients admitted to clinical wards without a requirement for critical care.23 We then quantified a panel of miRNAs previously associated with mechanistic pathways that could be altered in COVID-19 and demonstrated that specific circulating miRNA profiles are consistently associated with disease severity. Furthermore, we suggested that these represented a novel tool that could assist in the risk stratification of critically ill patients. A validation study in a large sample size is ongoing.
Since lung compartmentalization exists for multiple biological domains, an additional study was performed using respiratory samples.15 The same miRNA panel was quantified in bronchial aspirates from critically ill COVID-19 and non-COVID-19 patients, and we identified several individual miRNA ratios that predicted all-cause ICU mortality.15 In addition, we constructed a miRNA ratio-based prediction model for ICU mortality that showed an optimal discrimination value and outperformed the current best clinical predictor for ICU mortality.
Data managementEach sub-study followed a common iterative methodology agreed upon by the different parties. In each iteration, we first defined or revised the objectives, collected potentially relevant variables, and defined the sampling filters. Then, we selected the algorithms and methods for statistical analysis. Finally, we ran one or more experiments (based on different methods or their parameters) and generated detailed reports for experts to analyze and refine the objectives before starting a new iteration. Due to the iterative design, we prioritized having an automated process that could allow us to modify the least amount of code possible in each iteration (most commonly the revised set of relevant variables) and to generate interpretable detailed reports, regardless of the objectives, variables, and filters used in each sub-study. The processes of code validation, testing, database migration, and automated generation of different reports were integrated in a pipeline containing all stages needed to deliver a new version of the application. The automation of analysis allowed us to perform many sub-studies concurrently during the project. Nine of those were conducted through the automated analysis methodology, of which two have already been published17,24 and another two are under review with a journal. The remaining five are currently being drafted.
In the nine sub-studies, we produced 165.0 (12.0–370.0) univariate reports, 18.0 (6.0–30.0) multivariate analyses, and 5.0 (0.0–97.0) survival analyses, with a median of 28 propensity scores per sub-study. On average, it took 5.5 (3-11) months to obtain enough results to create a draft for a sub-study. This time included the evaluation and refinement of multiple medical hypothesis, as well as the production of the final figures and tables for publication.
Data qualityWe can summarize the results obtained by the DQA module as follows:
Unstructured text: These data include text with no structured format. Using a probability search algorithm, we converted the unstructured data to known tabulated data ready for processing by the data analytics module. We analyzed 30,566 unstructured texts from the 31,902 tabulated and validated texts (95.8%), discarding 542 and sending queries for 3.
Minimum data set (MDS) missing: This was the mandatory data to complete a sub-study. DQA analyzed 106,028 missing MDS and sent 623 queries, of which 326 were closed (52%).
Outliers: Data with values out of the statistical normal value range were queried in 4935 cases and resolved in 2427 (49%).
Inconsistent values: Conflicting values, like inconsistent dates or events. Inconsistent data could have more than one query because the incorrect value was not always clear. In total, 13,557 inconsistent values were queried, translating into 71,069 data queries. Currently, 19,329 data queries have been closed (27%).
DiscussionIn the present article, we described the method employed to carry-out a large epidemiological study, funded by ISCIII, to investigate patients with COVID-19 admitted to Spanish ICUs. We also describe some general patient data and study outputs.
Epidemiological studyWe included 6102 consecutive patients admitted to 55 ICUs homogeneously distributed throughout Spain, with a general data manager (AC) coordinating the following steps:
- 1.
Telephone contact of the principal investigator with the chief of the ICU.
- 2.
After acceptance, they tried to obtain approval from the ethics committee.
- 3.
The chief of the ICU appointed a person (or persons) to collect data for inclusion in the REDCAP database.
- 4.
ISCIII contracting for each data entry investigator.
- 5.
Some units preferred to be remunerated each time they collected data for a certain number of patients (e.g., each 100).
This process was certainly not easy and required diligence and a lot of energy. However, we learned the following lessons:
- 1.
The Spanish Health System was not prepared for automatic data collection from ICUs.
- 2.
ICUs use heterogeneous informatics systems that differ between regions and communities.
- 3.
Current law does not allow for rapid ethic approval in emergency epidemics such as COVID-19.
These issues meant that we did not publish our first manuscript until October 2021,24 contrasting with other European countries.1,2 However, we spent time and effort to maximize the amount of data with strict quality control, something that the first rapid publications could not achieve.
Overall, the crude in-hospital mortality was 31% in our population, similar or lower than reported elsewhere.1 More than two thirds of the patients needed invasive mechanical ventilation, with mortality increasing to 37% in this population. The remaining patients were mostly treated with high-flow nasal cannula, or to a lesser extent, with bilevel or continuous positive airway pressure. Intubated patients were mostly maintained in the prone position. In a subset of patients (Hospital Arnau de Vilanova), late intubation (>48h after ICU admission) was associated with higher mortality compared to early intubation. We are currently analyzing this finding in the overall population.
As shown in previous publications, patients admitted to ICU developed acute kidney injury, nosocomial infections, or pulmonary complications2-4. Of particular significance was the rate of nosocomial bacterial pneumonia (30%), which was associated with several worse outcomes.25
One of our goals was to determine the prognostic factors of the target population. For that reason, we collected data at hospital admission, ICU admission, day 3 after ICU admission, extubation, ICU discharge, and hospital discharge. This allowed the examination of prognostic factors for more than 1000 patients requiring mechanical ventilation. Age and increases in the ventilatory ratio and creatinine from day 1 to day 3 independently and significantly predicted in-hospital mortality.24 The ventilatory ratio, an easily calculated bedside parameter that uses PaCO2 and minute ventilation, is a surrogate for measuring lung dead space.5 Studies are ongoing into the impact of delayed intubation, non-invasive ventilatory support, driving pressure, COPD, a very elderly population, and the effectivity of corticosteroids and remdesivir.
Blood sample testingThe present research also collected 1068 samples from patients admitted to ICU. Importantly, these samples were obtained in the first 48hours after ICU admission, centralized in the IBSAL laboratory, and distributed to other labs. We also made significant efforts to obtain as much clinical data as possible relating to the patients from whom we had access to blood samples. The related studies published to date14,18 show that the viral RNA load, the positivity of antigen tests in blood, and the presence of low amounts of, or absent, specific antibodies were independently associated with disease severity and/or 30-day mortality. These findings are of key importance and could very easily be adapted for implementation in our National Health System. Indeed, our results indicate that two types of COVID-19 patient arrive at ICUs: poor and good viral controllers. These exhibit different biological responses and clinical outcomes, and they are currently being characterized by our group in an ongoing analysis of the entire CIBERESUCICOVID cohort.
Blood testing also looked at miRNAs, for which we demonstrated individual and collective potential to improve risk stratification in the critically ill patient. The host miRNA profiles linked to the disease and to fatal outcomes may allow a deeper understanding of the mechanistic pathways implicated in critical COVID-19 illness. Currently, we are validating these findings in a large population comprising more than 500 samples from different Spanish hospitals and we are analyzing the association between the circulating miRNA profile, pulmonary function, and radiologic features in survivors of critical COVID-19 illness. This study aims to provide novel insights into the pulmonary sequelae of COVID-19.
Follow-up studiesWe performed clinical follow-up studies in the overall population and in a cohort of patients from Hospital Arnau de Vilanova. In the overall cohort, age, ARDS, length of ICU stay, and ICU acquired nosocomial pneumonia were independently associated with long COVID syndrome at 3 months.26 In the Lleida cohort of patients with COVID-19 who were mechanically ventilated, 82% of patients who survived critical COVID-19 illness showed a lung diffusing capacity of less than 80% and abnormal chest CT scan in 70.2% at 3 months.27. In the overall population at 1 year follow-up, it was notable that the overall mortality of discharged patients was only 1%,17 a striking finding given that patients with community acquired pneumonia suffer mortality rates as high as 30%.28 Ongoing studies are considering the identification of clinical and biological predictors of long-term pulmonary sequelae.
We learned from our studies that patients discharged from ICU received care from several specialists and not intensivists. We have experiences difficulties in our efforts to contact these specialists, making it difficult to implement the follow-up protocol. This must be considered a limitation of the follow-up publications.
Data and quality managementThe integration of experts in medicine, data mining, and machine learning was a key challenge within the CIBERESUCICOVID project. On the one hand, we required a large number of medical hypotheses to be concurrently refined and tested, while on the other hand the different scientific practices of those disciplines demanded new definitions, formalizations, and automation of the research methodologies. Medical experts require certain statistical analyses for every data operation performed (e.g., univariate reports). This recurrent nature was recognized, and the process was automated, enabling certain sub-studies to produce in the order of thousands of statistical analyses. Data mining and machine learning methodologies, which may be unorthodox in medical practice, were suggested and integrated when possible (e.g., survival analysis or feature engineering). This enabled a distinct and heterogeneous approach to the problems and solutions posed by the pandemic, leading to a variety of sub-studies with differing complexities. At the same time, complex inconsistencies were automatically identified and classified with great precision. Synchronization with RedCap also worked efficiently to maintain real-time communication. However, we underestimated the many queries that would be generated, and to date, we have only debugged 65%, far from the projected 80%.
ConclusionsIn this manuscript, we present the methodology used in a large multicenter study sponsored by ISCIII to determine the short- and long-term outcomes in patients with COVID-19 admitted to more than 50 Spanish ICUs. This study included the collection of blood samples from more the 1000 patients. We have outlined the main results and messages of the published studies, the strengths and limitations of the study, and the ongoing or proposed analyses.
FundingFinancial support was provided by the Instituto de Salud Carlos III de Madrid (COV20/00110, ISCIII), Fondo Europeo de Desarrollo Regional (FEDER), “Una manera de hacer Europa,” and Centro de Investigación Biomedica En Red-Enfermedades Respiratorias (CIBERES). DdGC has received financial support from Instituto de Salud Carlos III (Miguel Servet 2020: CP20/00041), co-funded by the European Social Fund (ESF) “Investing in your future.”
Authors’ contributionsAT, AM, AC, JBM, DdGC, RP, MB, IZP and FB participated in protocol development, study design, study management, and data interpretation, and wrote the first draft of the report. The remaining authors critically reviewed the first draft of the report. The CIBERESUCICOVID consortium participated in data collection.
Conflict of interestsThe authors have disclosed that they do not have any conflicts of interest.
The authors are indebted to Maria Arguimbau, Raquel Campo, Natalia Jarillo, Javier Muñoz, Elisabeth Sancho, and Manuel Sánchez (†) for their extensive support in project management and article preparation. We also acknowledge the magnificent contribution of all the involved collaborators and data entries. This project would have been impossible without their help.