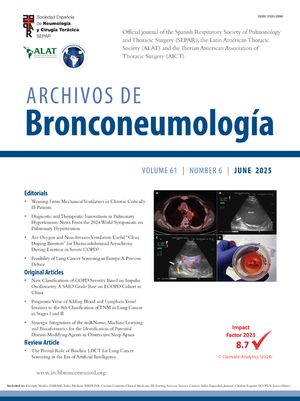
CURRENT SITUATION AND LONG-TERM CONSEQUENCES OF COVID-19 INFECTION
More infoConsidering the high variability of clinical course in the COVID-19, prognostic tools remain necessary for strategic healthcare planning. In hospitalized patients, clinical and demographic characteristics are associated with greater complications and death due to the disease.1–4 Chest computed tomography (CT) has also been widely used in the evaluation of these patients due to its high sensitivity,5 speed and relative availability, not only as a complementary diagnostic tool but also as an auxiliary method in clinical management and risk stratification.6,7 Therefore, the objective of our study is to analyze tomographic findings at admission of hospitalized patients with COVID-19 and their relationship with disease severity, using the need for invasive mechanical ventilation (MV) and in-hospital mortality as main outcomes.
We conducted a cross-sectional analysis of all confirmed COVID-19 cases in hospitalized adult patients, from January through December 2020, at a tertiary care public university referral hospital for COVID-19 care in Curitiba, the largest city in southern Brazil. We excluded patients who did not undergo chest CT or whose chest CT was performed outside the period of 5 days after hospital admission, as well as patients with incomplete data.
Chest CT was performed with high-resolution technique, with patients in a supine position and in complete inspiration, on a Toshiba Aquilion 64 CT scanner. The images were evaluated by certified radiologists. Patients were evaluated regarding the predominant tomographic pattern, which was considered to affect more than 50% of involved lung parenchyma. Patterns considered were ground-glass opacities, crazy paving (ground-glass opacities with intralobular septal thickening), consolidation and mixed pattern (half of the affected area with consolidation and the remainder with another tomographic pattern). Pulmonary artery diameter (PAD) and the dimensions of the mediastinal lymph nodes were evaluated in axial plane. Lymph nodes were considered enlarged when the smallest diameter found was greater than 10mm. The extent of lung parenchyma involvement was estimated according to visual scale in the axial plane and categorized into none, less than 25%, 25–50%, 50–75% and more than 75%. Clinical, laboratory, and demographic data were also collected.
Statistical analysis was performed using IBM SPSS Statistics software version 27. Quantitative variables were described in means±standard deviation or median (interquartile range [IQR]), as appropriate. Categorical variables were described as frequencies and percentages. The relationship of demographic, clinical and tomographic variables with mortality and the need for MV was evaluated using univariate logistic regression. A multivariate logistic regression was further performed to identify independent predictors. Only variables with at least 690 valid entries and with p values <0.05 in the univariate logistic regression analysis were included. The stepwise backward approach was used. Odds ratio (OR) with a 95% confidence interval (CI) were reported for all the variables analyzed. P values <0.05 were considered statistically significant.
In 2020, 1246 patients were hospitalized due to COVID-19 in our hospital, 492 of which were excluded from our analysis (Supplementary Fig. 1); thus, we assessed 754 patients. The mean age was 56.3±14.7 years old. The median time of symptoms onset until admission was 8 days (IQR 6–11). Clinical and demographic characteristics of the study population are shown in Supplementary Table 1.
Chest CT was performed within the first two days of hospitalization (median 0, IQR 0–1) in 86.7% of patients, with a median time between onset of symptoms and chest CT of 9 days (IQR 6–11). Similarly to data already reported,7–9 the most frequent finding was ground-glass opacity (53.9%), related to pulmonary alveolar oedema and partial filling of alveoli by proteinaceous exudate in the early stages of the disease10–12 (Table 1). As the disease progresses, with lymphocyte activation and release of inflammatory cytokines, these changes may become more extensive and there may be intra and interlobular septal infiltration with the presence of crazy paving, indicating both alveolar and interstitial involvement due to COVID-19.12,13 This pathophysiological mechanism seems to be related to the clinical worsening, which is in line with our findings, where crazy paving was found in 10.7% of our cases and, although not statistically significant regarding mortality in this analysis, was associated with more severe disease when compared to ground-glass opacities. Table 2 and Supplementary Table 2 show the characteristics of patients who died or needed MV, in comparison with those who did not.
Chest computed tomography results.
Chest CT characteristics | |||||
---|---|---|---|---|---|
Dominant pattern | N=736 | Lung involvement | N=749 | Other findings | N=754 |
Ground-glass opacity | 397 (53.9%) | None | 12 (1.6%) | Pleural effusion | 87 (11.5%) |
Consolidation | 53 (7.2%) | <25% | 122 (16.3%) | Lymph node enlargement | 158 (21.0%) |
Crazy paving | 81 (11%) | 25–50% | 239 (31.9%) | PADa (mm) | 27.6±4.2 |
Mixed | 205 (27.9%) | 50–75% | 215 (28.7%) | PAD>30mm | 167 (22.1%) |
>75% | 161 (21.5%) |
Laboratory abnormalities according to the degree of lung involvement in chest CT | |||||
---|---|---|---|---|---|
<25% | 25–50% | 50–75% | >75% | p* | |
Lymphocytesb | 134, 1152 (738–1766) | 238, 881 (565–1304) | 215, 850 (531–1200) | 161, 765 (533–1065) | <0.001 |
D-Dimerb | 121, 1.12 (0.52–4.05) | 217, 0.77 (0.45–1.44) | 207, 0.95 (0.54–1.79) | 157, 0.96 (0.6–2.09) | 0.009 |
Albuminc | 113, 3.63±0.59 | 193, 3.63±0.47 | 174, 3.57±0.44 | 137, 3.37±0.45 | <0.001 |
LDHb | 110, 269 (7–334) | 18, 333 (62–416) | 181, 384 (96–492) | 143, 420 (330–591) | <0.001 |
Ferritinb | 105, 453 (168–989) | 193, 830 (26–1527) | 189, 1144 (439–1675) | 159, 1547 (836–1675) | <0.001 |
C-reactive proteinb | 128, 2.54 (0.88–7.27) | 232, 6.06 (2.99–11.63) | 212, 7.86 (4.9–13.92) | 161, 12.36 (6.83–16) | <0.001 |
Procalcitoninb | 24, 0.13 (0.04–1.02) | 45, 0.1 (0.05–0.55) | 53, 0.11 (0.05–0.54) | 55, 0.15 (0.08–0.45) | 0.463 |
Pairwise comparison analysis of laboratory abnormalities according to the degree of lung involvement in chest CT | |||||
---|---|---|---|---|---|
Lymphocytesd | Albumind | C-reactive proteind | Ferritind | LDHd | |
<25% vs. 25-50% | <0.001 | 1 | <0.001 | 0.003 | 0.029 |
<25% vs. 50-75% | <0.001 | 1 | <0.001 | <0.001 | <0.001 |
<25% vs. >75% | <0.001 | <0.001 | <0.001 | <0.001 | <0.001 |
25–50% vs. 50–75% | 0.547 | 1 | 0.045 | 0.305 | 0.003 |
25––50% vs. >75% | 0.036 | <0.001 | <0.001 | <0.001 | <0.001 |
50–75% vs. >75% | 1 | 0.001 | 0.001 | 0.003 | 0.054 |
Relationship between pulmonary artery diameter (PAD) and laboratory abnormalities according to reference values | |||||
---|---|---|---|---|---|
Value (n) | PAD (mm)a | Value (n) | PAD (mm)a | P# | |
Lymphocytes (×103/μL) | ≥1000 (317) | 27.2±4.1 | <1000 (436) | 27.8±4.3 | 0.065 |
D-Dimer (mg/L FEU) | ≤0.55 (199) | 27.1±4.1 | >0.55 (507) | 27.9±4.3 | 0.023 |
Albumin (g/dL) | ≥3.5 (396) | 27.2±4.0 | <3.5 (225) | 28.3±4.5 | 0.001 |
Cardiac troponin (pg/ml) | <15.6 (435) | 27.1±3.8 | ≥15.6 (135) | 29.7±4.7 | <0.001 |
LDH (U/L) | <220 (61) | 27.2±3.1 | ≥220 (567) | 27.7±4.3 | 0.305 |
Ferritin (ng/mL) | ≤204 (74) | 27.9±4.5 | >204 (567) | 27.6±4.2 | 0.562 |
C-reactive protein (mg/dL) | ≤0.5 (28) | 27.6±3.6 | >0.5 (710) | 27.5±4.2 | 0.92 |
Procalcitonin (ng/mL) | <0.5 (132) | 27.4±4.3 | ≥0.5 (45) | 27.9±3.9 | 0.544 |
PAD: pulmonary artery diameter.
Data reported in n (%), except when indicated otherwise.
Factors associated with in-hospital death.
Clinical characteristics | N | Hospital Discharge | Death | OR | CI95% | p* |
---|---|---|---|---|---|---|
Age≥60 years old | 318 | 224 (70.4%) | 94 (29.6%) | 4.66 | 3.07–7.08 | <0.001 |
Male sex | 390 | 325 (83.3%) | 65 (16.7%) | 0.92 | 0.63–1.34 | 0.665 |
Smoking history | 177 | 134 (75.7%) | 43 (24.3%) | 2.15 | 1.36–3.39 | 0.001 |
Obesity | 224 | 194 (86.6%) | 30 (13.4%) | 0.66 | 0.43–1.03 | 0.07 |
Hypertension | 379 | 305 (80.5%) | 74 (19.5%) | 1.38 | 0.94–2.02 | 0.096 |
Diabetes Mellitus | 238 | 190 (79.8%) | 48 (20.2%) | 1.34 | 0.90–1.98 | 0.149 |
Coronary artery disease | 63 | 49 (77.8%) | 12 (22.2%) | 1.42 | 0.76–2.65 | 0.276 |
COPD | 48 | 34 (70.8%) | 14 (29.2%) | 2.09 | 1.09–4.03 | 0.027 |
Chronic heart disease | 132 | 101 (76.5%) | 31 (23.5%) | 1.62 | 1.03–2.56 | 0.038 |
Chronic lung disease | 110 | 85 (77.3%) | 25 (22.7%) | 1.51 | 0.92–2.47 | 0.101 |
Chronic liver disease | 8 | 3 (37.5%) | 5 (62.5%) | 8.28 | 1.95–35.1 | 0.004 |
Chronic kidney disease | 34 | 21 (61.8%) | 13 (38.2%) | 3.19 | 1.55–6.55 | 0.002 |
Active cancer | 33 | 21 (63.6%) | 12 (36.4%) | 2.92 | 1.40–6.10 | 0.004 |
Laboratory tests | N | Hospital discharge | Death | OR | 95% CI | p* |
---|---|---|---|---|---|---|
Lymphocytesa,b | 753 | 623, 910 (577–1327) | 130, 760 (462–1013) | 0.92 | 0.89–0.96 | <0.001 |
C-reactive proteinb | 738 | 608, 6.58 (2.91–12.68) | 130, 10.52 (5.54–15.62) | 1.08 | 1.04–1.12 | <0.001 |
Ferritina,b | 641 | 520, 900 (412–1675) | 121, 1210 (478–1675) | 1.04 | 1.003–1.07 | 0.031 |
LDHa,b | 628 | 511, 336 (263–428) | 117, 436 (319–593) | 1.4 | 1.26–1.55 | <0.001 |
d-Dimerb | 706 | 581, 0.84 (0.48–1.71) | 125, 1.44 (0.77–3.67) | 1.03 | 1.01–1.05 | 0.009 |
Albuminc | 621 | 507, 3.61±0.45 | 114, 3.32±0.60 | 0.32 | 0.21–0.49 | <0.001 |
Procalcitoninb | 177 | 104, 0.09 (0.05–0.24) | 73, 0.26 (0.08–1.35) | 1.27 | 1.01–1.59 | 0.039 |
Cardiac troponina,b | 570 | 455, 10 (10–10) | 115, 14.3 (10–61.40) | 1.01 | 0.99–1.03 | 0.394 |
Chest CT | N | Hospital discharge | Death | OR | 95% CI | p* |
---|---|---|---|---|---|---|
Lung involvement area | ||||||
≤75%d | 588 | 505 (85.9%) | 83 (14.1%) | |||
>75% | 161 | 114 (70.8%) | 47 (29.2%) | 2.51 | 1.66–3.78 | <0.001 |
Dominant pattern | ||||||
Ground-glass opacityd | 397 | 323 (81.4%) | 74 (18.6%) | |||
Mixed | 205 | 181 (88.3%) | 24 (11.7%) | 0.58 | 0.35–0.95 | 0.03 |
Crazy paving | 81 | 59 (72.8%) | 22 (27.2%) | 1.63 | 0.94–2.82 | 0.083 |
Consolidation | 53 | 45 (84.9%) | 8 (15.1%) | 0.78 | 0.35–1.72 | 0.531 |
Pleural effusion | 87 | 56 (64.4%) | 31 (35.6%) | 3.18 | 1.95–5.17 | <0.001 |
Lymph node enlargement | 158 | 120 (76.0%) | 38 (24.1%) | 1.73 | 1.13–2.66 | 0.011 |
PAD (mm)c | 754 | 624, 27.3±4.1 | 139, 28.9±4.6 | 1.09 | 1.04–1.13 | <0.001 |
Independent predictors of in-hospital mortality (multivariate analysis)e | |||
---|---|---|---|
OR | 95% CI | p* | |
Age≥60 years old | 4.38 | 2.76–6.95 | <0.001 |
Active cancer | 2.99 | 1.21–7.17 | 0.018 |
Chronic liver disease | 11.58 | 2.22–60.38 | 0.004 |
Lymphocytesa | 0.95 | 0.91–0.997 | 0.037 |
C-reactive protein | 1.05 | 1.002–1.09 | 0.038 |
Lung involvement>75% | 2.25 | 1.39–3.63 | <0.001 |
Pleural effusion | 2.36 | 1.33–4.19 | 0.003 |
Variables initially included in the model: age≥60 years old, chronic heart disease, chronic obstructive pulmonary disease, active cancer, chronic kidney disease, chronic liver disease, C-reactive protein, lymphocytes, d-dimer, lung parenchyma involvement >75%, pleural effusion and pulmonary artery diameter.
When assessing the extent of lung disease on chest CT, half of the patients had parenchyma involvement greater than 50%. Greater lung parenchyma involvement was significantly associated with high values of CRP, ferritin and LDH and low lymphocytes and albumin, probably indicating that these findings are related to more extensive involvement on CT. In addition, lung parenchyma involvement greater than 75% was independently associated with a higher risk of clinical worsening (OR=2.25, 95% CI 1.39–3.63, p<0.001) and death (OR=2.6, 95% CI 1.37–3.09, p<0.001). The relationship between the degree of lung parenchyma involvement, assessed by different scores, and worse outcomes for COVID-19 has also been described in other studies,8,9,11,14 which reinforces the role of chest CT as a prognostic tool for COVID-19.
Another independent predictor of mortality identified in chest CT was the presence of pleural effusion, found in 11.5% of patients, with an adjusted OR of 2.36 (95% CI 1.33–4.19, p=0.003) compared to patients without it. A similar result was found in a meta-analysis which reported a 9.5% prevalence of pleural effusion, with an OR of 4.53 (95% CI 2.16–9.49) for mortality in this group.15 This fact seems to be associated with direct injury to the lung tissue by SARS-CoV-2 and with a higher systemic inflammatory response,16 analogous to that already described in other viral respiratory infections, such as MERS17 and H1N1.18
In our cohort, patients who needed MV or who died from COVID-19 also had larger PAD. The mean PAD was 27.6±4.2mm and a diameter greater than 30mm was found in 167 patients (22.1%). Esposito et al. reported a relationship between PAD enlargement and in-hospital mortality due to COVID-19, even after adjustment for demographic characteristics and comorbidities, suggesting that this is an acute complication of the disease.19 The main mechanism related to larger PAD is related to increased pulmonary vascular resistance, secondary to endothelial injury, tissue inflammation and prothrombotic state with occlusion of small vessels.20 Although cardiovascular and thromboembolic complications were not evaluated in this study, patients with higher d-dimer and cardiac troponin presented significantly larger PAD when compared to patients with normal values (27.1±4.1mm vs. 27.9±4.3, p=0.023; and 27.1±3.8 vs. 29.7±4.7, p<0.001, respectively).
This study has limitations. The collection of data from medical records led to the exclusion of patients due to incomplete data since care was provided without a standardized protocol of data collection. Another limitation is that all patients were admitted to the same public hospital, so data may not accurately represent the reality of other regions.
In conclusion, easily obtainable chest CT data, such as extent of lung involvement in visual scale, PAD and presence of pleural effusion, can be used as predictors of disease severity and in-hospital mortality in COVID-19.
Ethics approvalThe study was previously approved by the local Research Ethics Committee, under the opinion 4.215.032, of August 16th, 2020, with waiver of informed consent.
FundingThis research did not receive any specific grant from funding agencies in the public, commercial, or not-for-profit sectors.
Data availability statementThe datasets generated and analyzed during the current study are available from the corresponding author on reasonable request.
Conflict of interestNone declared.
The authors would like to thank all professionals involved in the care of patients with COVID-19, as well as patients themselves and their families.