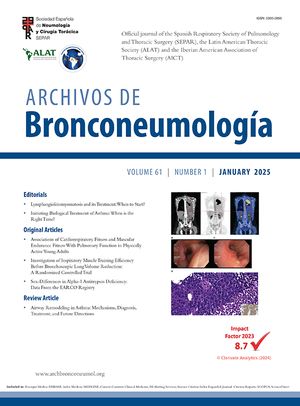
Lung cancer remains the leading cause of cancer-related deaths worldwide, mainly due to late diagnosis and the presence of metastases. Several countries around the world have adopted nation-wide LDCT-based lung cancer screening that will benefit patients, shifting the stage at diagnosis to earlier stages with more therapeutic options. Biomarkers can help to optimize the screening process, as well as refine the TNM stratification of lung cancer patients, providing information regarding prognostics and recommending management strategies. Moreover, novel adjuvant strategies will clearly benefit from previous knowledge of the potential aggressiveness and biological traits of a given early-stage surgically resected tumor. This review focuses on proteins as promising biomarkers in the context of lung cancer screening. Despite great efforts, there are still no successful examples of biomarkers in lung cancer that have reached the clinics to be used in early detection and early management. Thus, the field of biomarkers in early lung cancer remains an evident unmet need.
A more specific objective of this review is to present an up-to-date technical assessment of the potential use of protein biomarkers in early lung cancer detection and management. We provide an overview regarding the benefits, challenges, pitfalls and constraints in the development process of protein-based biomarkers. Additionally, we examine how a number of emerging protein analytical technologies may contribute to the optimization of novel robust biomarkers for screening and effective management of lung cancer.