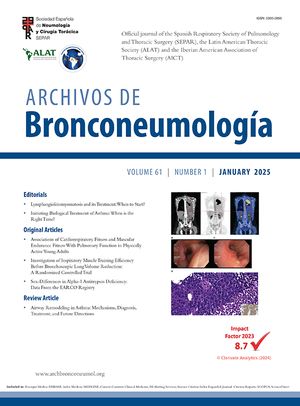
Lung cancer is the leading cause of cancer death and the second most common cancer in both sexes worldwide, with tobacco being its main risk factor. The aim of this study is to establish the temporal relationship between smoking prevalence and lung cancer mortality in Spain.
MethodsTo model the time dependence between smoking prevalence and lung cancer mortality, a distributed lag non-linear model was applied adjusting for sex, age, year of mortality and population at risk. Smoking prevalence data from 1991-2020 were used. Considering a maximum lag of 25 years, mortality data from 2016-2020 were included. The effect of prevalence on mortality for each lag is presented in terms of relative risk (RR). To identify the lag at which smoking prevalence has the greatest effect on mortality, the RR of the different lags were compared.
ResultsThe optimal lag observed between smoking prevalence and lung cancer mortality in Spain was 15 years. The maximum RR was 2.9 (95%CI: 2.0-4.3) for a prevalence of 71% and a 15-year lag. The RR was 1.8 for a prevalence of 33%, an approximate median value between 1991-2020, and a 15-year lag.
ConclusionsIn Spain, lung cancer mortality is affected by smoking prevalence 15 years prior. Knowing the evolution of the smoking prevalence series in a country and establishing a lag time is essential to predict how lung cancer incidence and mortality will evolve.